How Big Data Transforms Decision Making
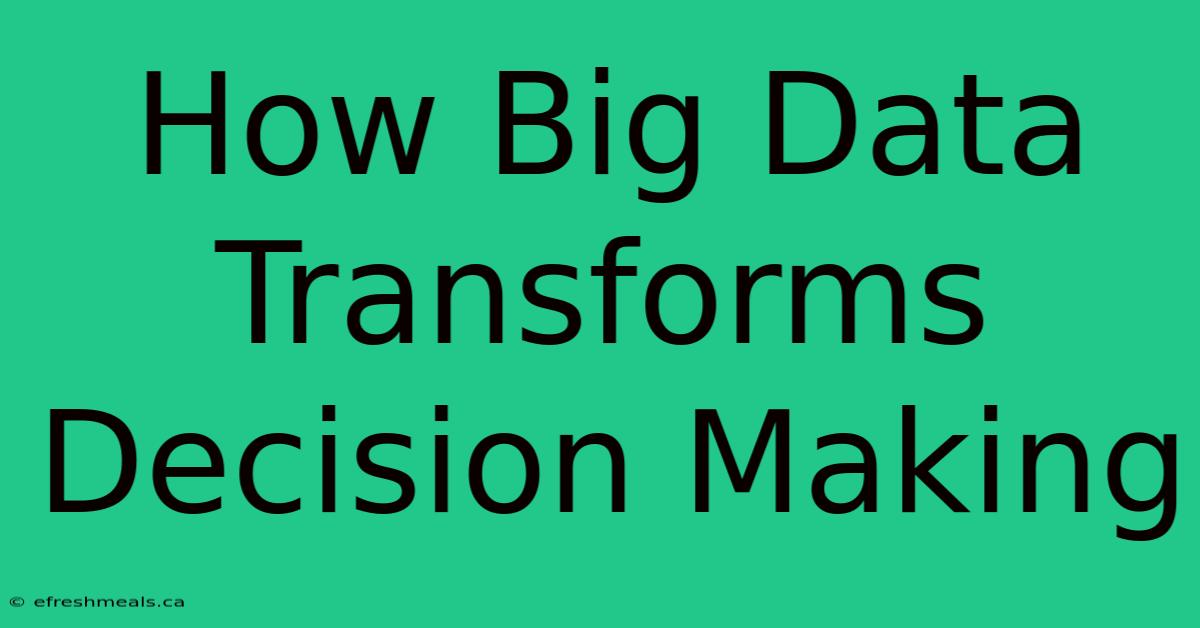
Discover more detailed and exciting information on our website. Click the link below to start your adventure: Visit Best Website nimila.me. Don't miss out!
Table of Contents
How Big Data Transforms Decision Making: Unveiling Powerful Insights
Editor's Note: Big data analytics is revolutionizing how businesses and organizations make decisions. This article explores how this transformative technology provides unprecedented insights, leading to more effective strategies and improved outcomes.
Why It Matters
Big data, the massive volume of structured and unstructured data generated daily, presents both challenges and immense opportunities. Understanding how to harness this data effectively is crucial for success in today's competitive landscape. This review delves into the key ways big data analytics improves decision-making across various sectors, highlighting its impact on strategic planning, risk management, and operational efficiency. Related keywords include data analytics, business intelligence, predictive modeling, machine learning, data visualization, and decision support systems.
Key Takeaways of Big Data Analytics
Benefit | Description |
---|---|
Improved Accuracy | Data-driven insights reduce reliance on gut feelings and assumptions. |
Enhanced Efficiency | Automation streamlines processes, freeing up time for strategic initiatives. |
Predictive Capabilities | Forecasting future trends enables proactive planning and resource allocation. |
Personalized Experiences | Tailored offerings based on individual customer preferences enhance satisfaction. |
Reduced Risks | Identifying and mitigating potential problems early improves outcomes. |
How Big Data Transforms Decision Making
Big data analytics empowers organizations to move beyond intuition and anecdotal evidence, relying instead on concrete data to inform strategic decisions. This shift facilitates more accurate predictions, optimized resource allocation, and ultimately, improved outcomes.
Data-Driven Insights: The Foundation of Effective Decision Making
Big data's transformative power stems from its ability to provide comprehensive and detailed insights. By analyzing vast datasets, organizations can uncover hidden patterns, trends, and correlations that would be impossible to identify using traditional methods. This granular level of understanding allows for more precise and targeted interventions. For example, a retail company can analyze customer purchase history to personalize marketing campaigns, leading to increased sales and customer loyalty.
Predictive Modeling: Forecasting Future Trends
Predictive modeling leverages historical data to forecast future outcomes. By identifying patterns and trends, businesses can anticipate market changes, customer behavior, and potential risks. This proactive approach allows for strategic adjustments, minimizing negative impacts and maximizing opportunities. For instance, a financial institution might use predictive modeling to identify individuals at high risk of defaulting on loans, enabling preventative measures.
Real-time Analytics: Adapting to Dynamic Environments
Real-time analytics allows organizations to respond quickly to changing circumstances. By processing data as it's generated, businesses can make immediate adjustments to optimize operations and improve customer experiences. This responsiveness is particularly critical in industries where rapid change is the norm, such as finance and e-commerce. For example, a transportation company might use real-time data to adjust routes based on traffic conditions, improving efficiency and reducing delays.
The Relationship Between Risk Management and Big Data Analytics
Introduction
Effective risk management is paramount for organizational success. Big data analytics provides crucial tools to identify, assess, and mitigate potential risks more effectively than traditional methods. By analyzing vast datasets, organizations can detect patterns and anomalies that indicate potential problems before they escalate.
Facets of Risk Management with Big Data
- Identification: Big data enables the identification of previously unseen risk factors through pattern recognition and anomaly detection.
- Assessment: Quantitative analysis of data allows for a more precise assessment of the likelihood and potential impact of risks.
- Mitigation: Data-driven insights guide the development of effective strategies to mitigate identified risks.
- Monitoring: Continuous monitoring of relevant data allows for proactive identification and response to emerging risks.
- Reporting: Big data facilitates the creation of comprehensive and detailed risk reports for stakeholders.
Summary
The integration of big data analytics into risk management enhances the effectiveness and efficiency of risk mitigation efforts, leading to better overall organizational resilience.
The Interplay Between Operational Efficiency and Big Data Analytics
Introduction
Operational efficiency is a critical driver of profitability and competitiveness. Big data analytics plays a significant role in optimizing various operational aspects. By analyzing operational data, businesses can identify bottlenecks, streamline processes, and improve resource allocation.
Further Analysis
Consider a manufacturing company that uses sensor data from its machinery to predict potential equipment failures. By identifying patterns indicative of impending malfunctions, the company can schedule preventative maintenance, minimizing downtime and maximizing production efficiency.
Closing
Big data empowers organizations to achieve significant improvements in operational efficiency. Through data-driven insights, companies can enhance productivity, reduce costs, and improve overall performance. The challenge lies in implementing robust data collection and analysis systems capable of handling the sheer volume and complexity of modern datasets.
Information Table: Key Benefits of Big Data in Decision Making
Area | Benefit | Example |
---|---|---|
Marketing | Personalized campaigns, improved customer targeting | Tailored email marketing based on individual customer preferences |
Sales | Improved forecasting, optimized pricing strategies | Predicting sales based on seasonal trends and economic indicators |
Operations | Streamlined processes, reduced waste, improved efficiency | Predictive maintenance to minimize downtime in manufacturing |
Risk Management | Early identification and mitigation of potential risks | Fraud detection in financial transactions |
Product Development | Improved understanding of customer needs, faster innovation | Developing new products based on customer feedback and market research data |
Customer Service | Faster resolution of issues, improved customer satisfaction | Chatbots providing immediate answers to frequently asked questions |
FAQ
Introduction
This section addresses common questions about the role of big data in decision-making.
Questions
- Q: What types of data are used in big data analytics? A: Big data analytics uses both structured (e.g., databases) and unstructured (e.g., social media posts, images) data.
- Q: How can I implement big data analytics in my organization? A: Start by identifying key business problems, selecting appropriate data sources, and choosing the right analytics tools.
- Q: What are the challenges of using big data analytics? A: Challenges include data security, data quality, and the need for skilled personnel.
- Q: Is big data analytics suitable for small businesses? A: Yes, even small businesses can benefit from big data analytics using cloud-based solutions.
- Q: What are the ethical considerations of big data analytics? A: Issues like data privacy and bias in algorithms need careful consideration.
- Q: How can I ensure the accuracy of big data insights? A: Data quality, robust analytical methods, and validation processes are crucial.
Summary
The FAQ section highlights the practical aspects of implementing big data analytics, addressing both opportunities and challenges.
Tips for Effective Big Data Decision Making
Introduction
This section offers practical advice for leveraging big data effectively in decision-making processes.
Tips
- Clearly Define Your Objectives: Identify the specific business questions you want to answer with big data.
- Ensure Data Quality: Invest in data cleansing and validation to ensure accuracy and reliability.
- Choose the Right Tools: Select analytics tools appropriate for your data volume and complexity.
- Develop a Data-Driven Culture: Foster a company-wide understanding and acceptance of data-driven decision-making.
- Visualize Your Data: Use data visualization tools to communicate insights effectively to stakeholders.
- Continuously Monitor and Evaluate: Track the impact of your data-driven decisions and refine your approach accordingly.
- Prioritize Data Security and Privacy: Implement robust security measures to protect sensitive data.
- Invest in Skilled Personnel: Hire or train individuals with expertise in big data analytics.
Summary
Following these tips can significantly improve the effectiveness of big data in decision-making, leading to more informed choices and better outcomes.
Summary of How Big Data Transforms Decision Making
This article explored the transformative role of big data analytics in decision-making. From improving accuracy and efficiency to enabling predictive modeling and real-time analysis, big data provides organizations with unparalleled insights. Understanding how to leverage this powerful tool is key to staying competitive and achieving business success in today's data-driven world.
Closing Message
The future of decision-making lies in the effective utilization of big data. By embracing data-driven insights and investing in the necessary technologies and expertise, organizations can unlock unprecedented opportunities and achieve sustainable growth. The journey towards data-driven decision-making requires commitment, careful planning, and a willingness to adapt to the ever-evolving landscape of big data analytics.
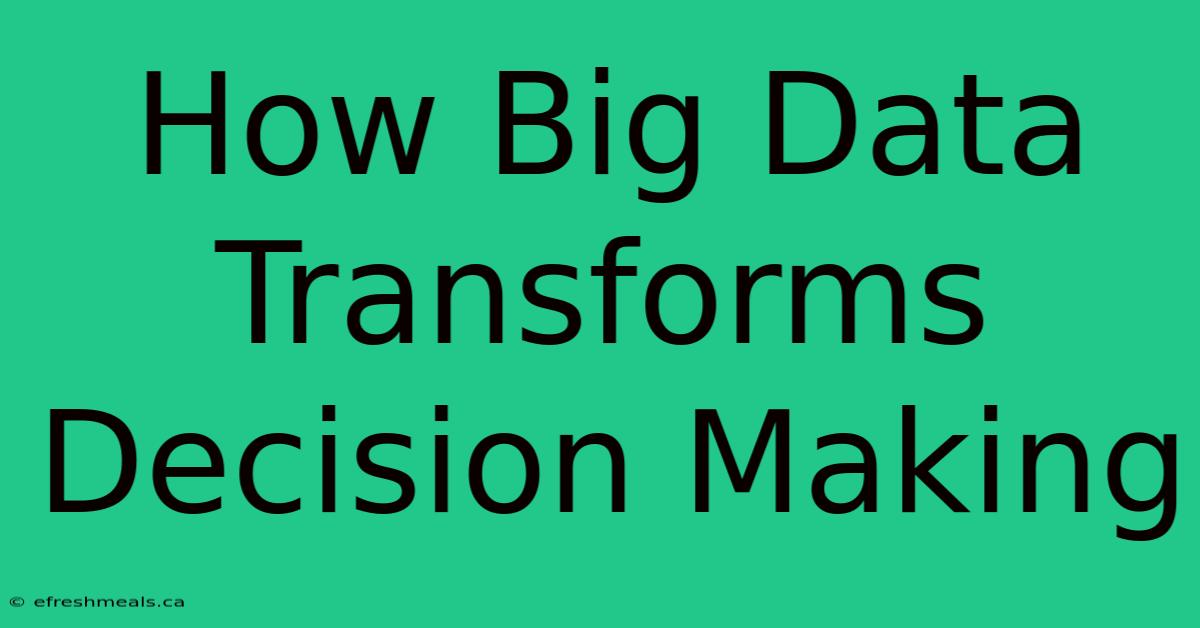
Thank you for visiting our website wich cover about How Big Data Transforms Decision Making. We hope the information provided has been useful to you. Feel free to contact us if you have any questions or need further assistance. See you next time and dont miss to bookmark.
Featured Posts
-
Aleppo Breached Syrian Rebels Launch Shock Attack
Nov 30, 2024
-
Best Le Creuset Black Friday Deals
Nov 30, 2024
-
Survivor Series War Games 2024 Complete Guide
Nov 30, 2024
-
Canucks Overcome Sabres Garland Scores
Nov 30, 2024
-
Mexican Diva Silvia Pinal Passes Away At 93
Nov 30, 2024