Top 5 Data Analytics For Indian Businesses
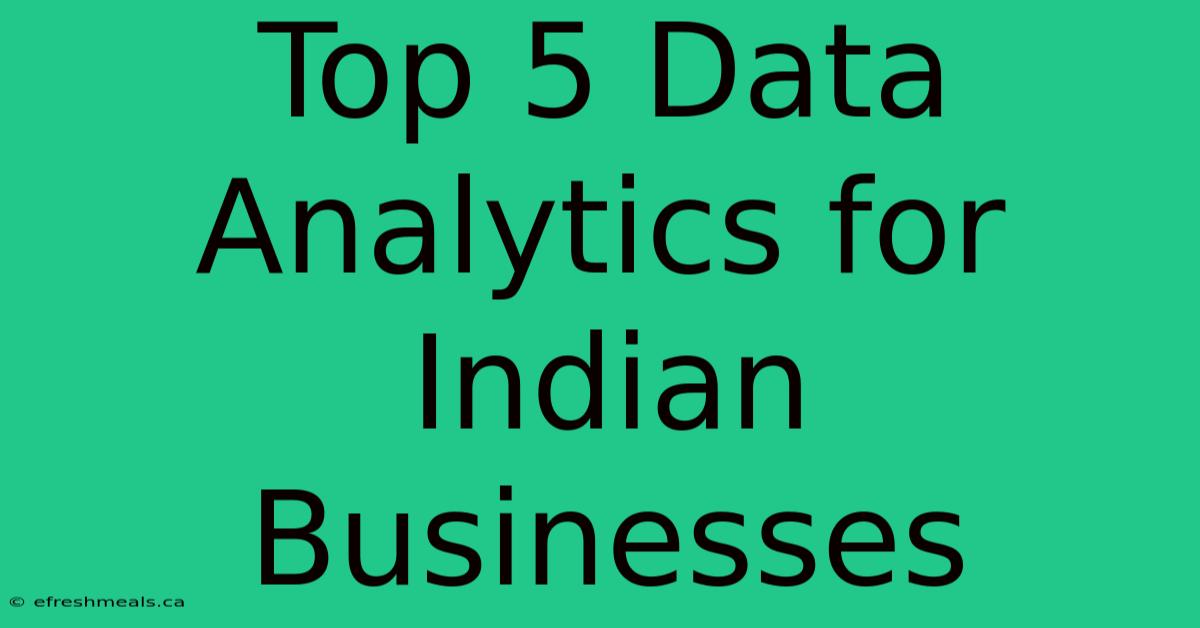
Discover more detailed and exciting information on our website. Click the link below to start your adventure: Visit Best Website nimila.me. Don't miss out!
Table of Contents
Top 5 Data Analytics Techniques Transforming Indian Businesses
Editor's Note: Unlocking the potential of data analytics is no longer optional for Indian businesses. This article reveals five crucial techniques driving growth and efficiency.
Why Data Analytics Matters for Indian Businesses
The Indian business landscape is dynamic and competitive. Understanding market trends, customer behavior, and operational efficiency is critical for success. Data analytics provides the insights needed to make informed decisions, optimize processes, and gain a competitive edge. This review explores five key data analytics techniques—predictive modeling, customer segmentation, sentiment analysis, web analytics, and supply chain optimization—and their impact on Indian businesses across various sectors. Related keywords include business intelligence, data-driven decision-making, market research, and competitive advantage.
Key Takeaways of Data Analytics for Indian Businesses
Technique | Benefit | Example in Indian Context |
---|---|---|
Predictive Modeling | Forecasts future trends & customer behavior | Predicting demand for agricultural products |
Customer Segmentation | Targets specific customer groups effectively | Personalizing offers for different age groups |
Sentiment Analysis | Understands customer opinions & feedback | Analyzing social media comments on new products |
Web Analytics | Optimizes website performance & user experience | Improving e-commerce website conversion rates |
Supply Chain Optimization | Streamlines logistics & reduces costs | Optimizing delivery routes for e-commerce firms |
Top 5 Data Analytics Techniques for Indian Businesses
Predictive Modeling
Introduction: Predictive modeling uses historical data and statistical algorithms to forecast future outcomes. This is invaluable for anticipating market trends, managing risks, and optimizing resource allocation.
Key Aspects: Predictive modeling involves data cleaning, feature engineering, model selection (regression, classification, time series), model evaluation, and deployment. Different models suit different business problems.
Discussion: In India, predictive modeling can be applied to diverse sectors. For example, a financial institution can use it to predict loan defaults, while an e-commerce company can use it to forecast product demand. The accuracy of predictions depends on data quality and the chosen model.
Customer Segmentation
Introduction: Customer segmentation divides customers into groups based on shared characteristics (demographics, behavior, etc.). This allows for targeted marketing campaigns and personalized experiences.
Facets: Segmentation techniques include RFM analysis (recency, frequency, monetary value), cluster analysis, and cohort analysis. Each technique has strengths and weaknesses depending on the data available and business goals. Risks include biased data leading to inaccurate segmentation, and the need for ongoing refinement as customer behavior changes. Mitigation strategies involve using robust data cleaning and validation techniques and regularly reviewing and updating segmentation models. Impacts include improved customer retention, increased sales, and more efficient marketing spend.
Summary: Effective customer segmentation is crucial for maximizing ROI on marketing efforts and fostering customer loyalty.
Sentiment Analysis
Introduction: Sentiment analysis gauges public opinion towards a brand, product, or service by analyzing textual data (social media posts, reviews, etc.).
Further Analysis: This technique helps companies understand customer perceptions, identify areas for improvement, and manage their brand reputation. For instance, analyzing customer reviews on e-commerce platforms can reveal insights into product strengths and weaknesses. Analyzing social media mentions can provide real-time feedback on marketing campaigns and brand perception. Challenges include handling sarcasm and nuanced language, and the need for large datasets for accurate results.
Closing: Sentiment analysis provides invaluable feedback, allowing businesses to adapt and improve based on actual customer feelings.
Web Analytics
Introduction: Web analytics tracks website traffic and user behavior to understand website effectiveness and optimize user experience.
Facets: Key metrics include bounce rate, conversion rate, time on site, and traffic sources. Tools like Google Analytics provide comprehensive data. Risks include inaccurate tracking if implemented incorrectly and the need for regular monitoring and adjustments. Mitigation involves employing proper tracking methods and regularly reviewing the data to identify potential issues. Impacts include improved website design, targeted marketing campaigns, and enhanced customer engagement.
Summary: Web analytics empowers businesses to improve their online presence and enhance customer engagement.
Supply Chain Optimization
Introduction: Supply chain optimization uses data to improve efficiency and reduce costs throughout the supply chain.
Further Analysis: This involves analyzing data from various sources (inventory levels, transportation costs, supplier performance) to identify bottlenecks and areas for improvement. Techniques include forecasting demand, optimizing inventory levels, and improving logistics. Examples in India include optimizing delivery routes for e-commerce businesses or improving the efficiency of agricultural supply chains. Challenges include integrating data from different sources and managing the complexity of large supply chains.
Closing: Efficient supply chain management is critical for profitability and competitiveness in today's globalized market.
Key Insights Table: Data Analytics for Indian Businesses
Technique | Key Metric(s) | Benefits | Challenges |
---|---|---|---|
Predictive Modeling | Accuracy, precision, recall | Improved forecasting, risk management | Data quality, model complexity |
Customer Segmentation | Customer lifetime value, ROI | Targeted marketing, personalized offers | Data privacy, segmentation accuracy |
Sentiment Analysis | Positive/negative sentiment scores | Brand reputation management, feedback | Handling nuanced language, data volume |
Web Analytics | Conversion rate, bounce rate | Website optimization, user experience | Accurate tracking, data interpretation |
Supply Chain Optimization | Inventory turnover, delivery time | Cost reduction, efficiency improvement | Data integration, supply chain complexity |
FAQ
Introduction: This section answers common questions about data analytics for Indian businesses.
Questions:
- Q: What type of data is most useful for Indian businesses? A: A variety of data types are useful, including transactional data, customer data, market research data, and social media data.
- Q: How much does data analytics cost? A: Costs vary depending on the techniques used, the size of the data, and the level of expertise required.
- Q: What are the ethical considerations of using data analytics? A: Data privacy and security are crucial. Adherence to regulations like GDPR and local Indian laws is vital.
- Q: What skills are needed for effective data analytics? A: Skills range from data manipulation and analysis to statistical modeling and data visualization.
- Q: Are there any free data analytics tools available? A: Yes, there are many free tools available, including Google Analytics and open-source software.
- Q: How can small businesses in India benefit from data analytics? A: Even small businesses can benefit from basic analytics techniques, such as tracking website traffic and analyzing customer feedback.
Summary: Addressing these FAQs highlights the accessibility and importance of data analytics, regardless of business size or resources.
Tips for Implementing Data Analytics in Indian Businesses
Introduction: This section offers practical tips for successfully implementing data analytics.
Tips:
- Define clear business objectives: Start with specific goals for what you want to achieve with data analytics.
- Invest in data infrastructure: Ensure you have the necessary hardware, software, and data storage capabilities.
- Build a skilled team: Recruit or train employees with the necessary data analysis skills.
- Prioritize data quality: Accurate, clean data is crucial for effective analysis.
- Start small and iterate: Begin with a pilot project to test different techniques before scaling up.
- Use visualization tools: Present data insights in a clear and understandable way to communicate effectively with stakeholders.
- Continuously monitor and improve: Regularly review your data analysis processes and make adjustments as needed.
Summary: These tips provide a roadmap for successful data-driven decision-making.
Summary of Top 5 Data Analytics Techniques for Indian Businesses
This article explored five key data analytics techniques – predictive modeling, customer segmentation, sentiment analysis, web analytics, and supply chain optimization – and their transformative potential for Indian businesses. Understanding and implementing these techniques can lead to significant improvements in efficiency, profitability, and competitive advantage. The insights presented offer a practical framework for harnessing the power of data to drive growth and success in the dynamic Indian market.
Closing Message: Embracing data analytics isn't just a trend; it's a necessity for survival and thriving in today's competitive landscape. Begin your data-driven journey today.
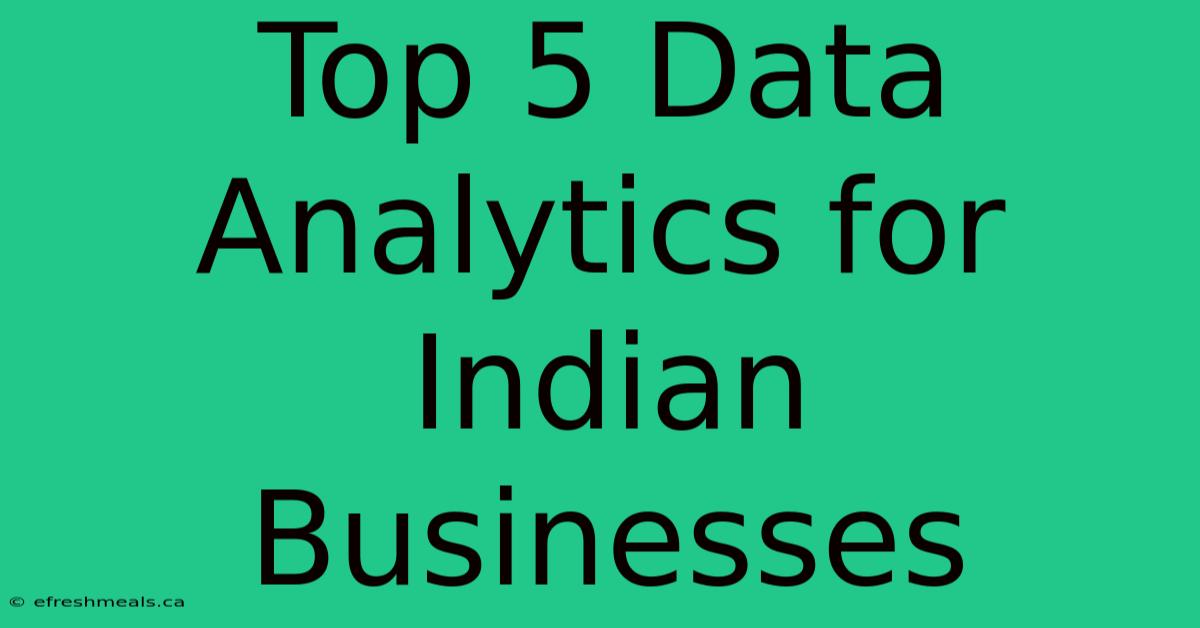
Thank you for visiting our website wich cover about Top 5 Data Analytics For Indian Businesses. We hope the information provided has been useful to you. Feel free to contact us if you have any questions or need further assistance. See you next time and dont miss to bookmark.
Featured Posts
-
Notre Dame Reopening Macrons Hope
Nov 30, 2024
-
Black Friday 2023 Lower Sales Expected
Nov 30, 2024
-
Amazon Canada Black Friday I Phone Deals
Nov 30, 2024
-
Bank Of Canada Forecast Misses 1 Growth
Nov 30, 2024
-
Aleppos Fall Insurgents Second City Breach
Nov 30, 2024