Turning Big Data Into Business Value
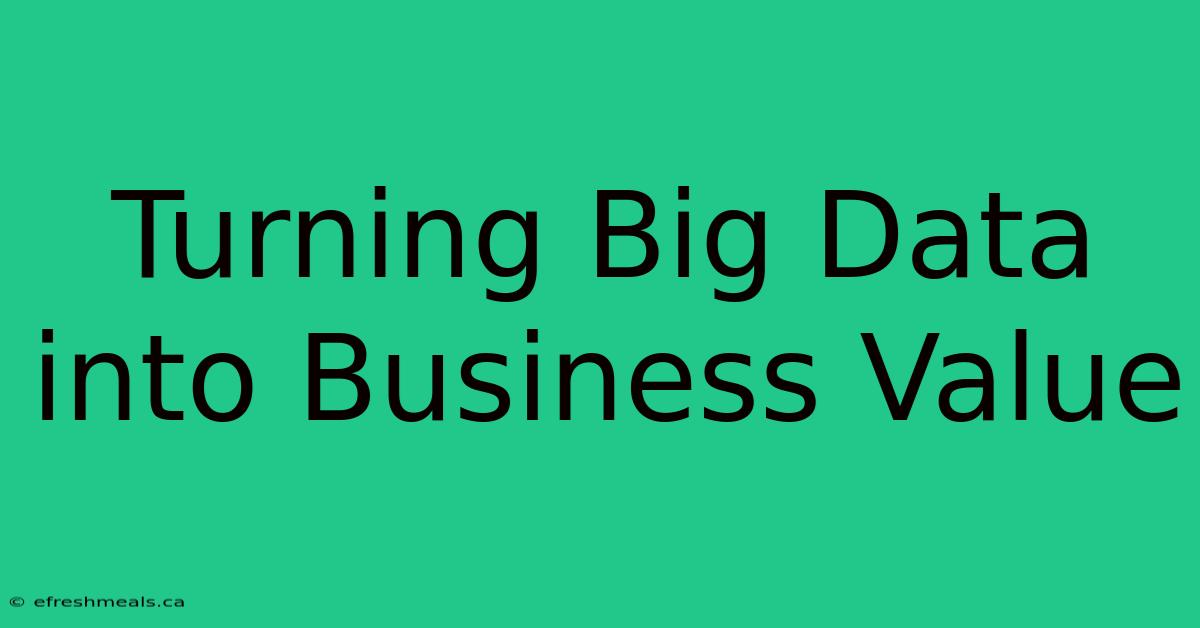
Discover more detailed and exciting information on our website. Click the link below to start your adventure: Visit Best Website nimila.me. Don't miss out!
Table of Contents
Turning Big Data into Business Value: Unveiling Hidden Insights
Editor's Note: The era of big data is upon us, but are businesses truly leveraging its potential? This article explores how to transform raw data into tangible business value.
Why It Matters
The sheer volume of data generated daily presents both a challenge and an opportunity. Understanding how to effectively analyze and interpret this information is crucial for competitive advantage. This article reviews key strategies and techniques for extracting actionable insights from big data, focusing on areas like improved decision-making, enhanced customer experiences, and optimized operational efficiency. Related keywords include: data analytics, business intelligence, data-driven decision making, predictive analytics, and data visualization.
Key Takeaways of Big Data Value
Takeaway | Description |
---|---|
Improved Decision-Making | Data-driven insights replace gut feelings, leading to more informed choices. |
Enhanced Customer Experience | Personalized offerings and targeted marketing campaigns boost customer satisfaction. |
Optimized Operational Efficiency | Streamlined processes and reduced waste through predictive maintenance and resource allocation. |
New Revenue Streams | Identifying new market opportunities and developing innovative products/services. |
Competitive Advantage | Gaining a clearer understanding of market trends and customer behaviors. |
Turning Big Data into Business Value
Introduction
Harnessing the power of big data requires a strategic approach encompassing data collection, storage, processing, analysis, and visualization. The key lies in transforming raw data into actionable intelligence that directly impacts the bottom line.
Key Aspects
- Data Collection: Gathering relevant data from various sources (internal systems, external databases, social media, etc.).
- Data Storage: Utilizing robust and scalable solutions to manage vast datasets efficiently.
- Data Processing: Cleaning, transforming, and preparing data for analysis using tools like ETL (Extract, Transform, Load).
- Data Analysis: Applying various analytical techniques (descriptive, diagnostic, predictive, prescriptive) to extract meaningful insights.
- Data Visualization: Presenting findings in a clear and concise manner using dashboards and reports to facilitate decision-making.
Data Analysis Techniques: Unlocking Business Insights
Introduction
Data analysis forms the core of turning big data into value. Different techniques cater to various business needs.
Facets of Data Analysis
-
Descriptive Analytics: Summarizing past data to understand trends and patterns (e.g., sales reports, website traffic). Role: Provides a historical context. Example: Analyzing website bounce rates to identify areas for improvement. Risk: Focusing solely on the past, neglecting future trends. Mitigation: Combining with predictive analytics. Impact: Improved understanding of current performance.
-
Diagnostic Analytics: Investigating the cause of observed trends and patterns (e.g., root cause analysis of customer churn). Role: Explains "why." Example: Determining why customer satisfaction scores dropped. Risk: Overlooking systemic issues. Mitigation: Utilizing multiple data sources. Impact: Enhanced problem-solving capabilities.
-
Predictive Analytics: Forecasting future outcomes based on historical data and statistical modeling (e.g., sales forecasting, risk management). Role: Anticipates future events. Example: Predicting customer lifetime value. Risk: Inaccurate models due to biased data. Mitigation: Data validation and model testing. Impact: Proactive decision-making.
-
Prescriptive Analytics: Recommending actions to optimize outcomes based on predictions and simulations (e.g., inventory optimization, personalized recommendations). Role: Guides actions. Example: Suggesting optimal pricing strategies. Risk: Overreliance on algorithms. Mitigation: Human oversight and interpretation. Impact: Optimized resource allocation.
Summary
Effective data analysis hinges on selecting the right technique based on the specific business question. By leveraging a combination of these methods, businesses can gain a comprehensive understanding of their operations and make well-informed decisions.
The Role of Data Visualization in Communicating Insights
Introduction
Even the most sophisticated analysis is useless if it cannot be effectively communicated. Data visualization plays a critical role in turning complex data into easily digestible insights.
Further Analysis
Effective data visualization uses charts, graphs, and dashboards to present key findings in a compelling and accessible manner. This ensures that stakeholders at all levels can understand the implications of the data and contribute to decision-making. Examples include heat maps to visualize customer churn rates, line graphs to track sales performance over time, and interactive dashboards to monitor key performance indicators (KPIs).
Closing
Data visualization is not just about aesthetics; it’s about clarity and communication. By choosing the right visualization tools and techniques, businesses can ensure that data-driven insights are accessible and actionable for everyone involved.
Key Insights Table: Transforming Data into Action
Data Source | Analysis Technique | Insight Gained | Business Action |
---|---|---|---|
Customer Surveys | Descriptive Analytics | Customer satisfaction trends | Identify areas for improvement in customer service. |
Sales Data | Predictive Analytics | Future sales projections | Optimize inventory levels and marketing campaigns. |
Website Analytics | Diagnostic Analytics | Reasons for high bounce rates | Improve website design and user experience. |
Social Media Data | Prescriptive Analytics | Optimal social media posting schedule | Maximize engagement and reach on social media. |
FAQ
Introduction
This section addresses common questions surrounding big data and its business applications.
Questions
-
Q: What are the challenges of using big data? A: Data volume, velocity, variety, veracity, and variability (the five Vs) pose significant challenges. Security and privacy concerns are also crucial.
-
Q: How much does it cost to implement a big data solution? A: Costs vary significantly depending on data volume, complexity, and required infrastructure.
-
Q: What skills are needed to work with big data? A: Data scientists, analysts, engineers, and database administrators are all crucial roles.
-
Q: What are some common big data tools? A: Hadoop, Spark, and cloud-based data warehousing solutions are widely used.
-
Q: How can I ensure data accuracy and reliability? A: Data governance, validation, and quality control processes are essential.
-
Q: How do I start implementing big data in my business? A: Begin by identifying key business questions and selecting relevant data sources.
Summary
Addressing these FAQs clarifies the process and challenges associated with big data implementation.
Tips for Turning Big Data into Business Value
Introduction
These tips offer practical steps to maximize the value derived from big data.
Tips
- Clearly Define Business Objectives: Start with specific questions you want answered.
- Invest in Data Infrastructure: Ensure you have the appropriate hardware and software.
- Build a Skilled Team: Assemble a team with expertise in data science and analytics.
- Prioritize Data Quality: Accurate and reliable data is crucial for meaningful insights.
- Use Data Visualization Effectively: Communicate your findings clearly and concisely.
- Continuously Monitor and Improve: Big data analysis is an iterative process.
- Embrace Collaboration: Work across departments to leverage data insights effectively.
- Stay Updated on Industry Trends: Big data technologies and techniques are constantly evolving.
Summary
By following these tips, businesses can effectively leverage big data to achieve significant business outcomes.
Summary of Turning Big Data into Business Value
This article explored the multifaceted process of transforming raw data into tangible business value. Key insights included the crucial roles of data analysis techniques, data visualization, and effective communication. By strategically collecting, processing, and analyzing big data, businesses can gain competitive advantages, enhance customer experiences, and optimize operational efficiency.
Closing Thoughts
The journey of turning big data into business value is an ongoing process of learning, adaptation, and innovation. Embracing a data-driven culture and continuously seeking new ways to leverage the power of data is key to staying ahead in today's competitive landscape. The future of business is inextricably linked to the ability to effectively harness the power of big data.
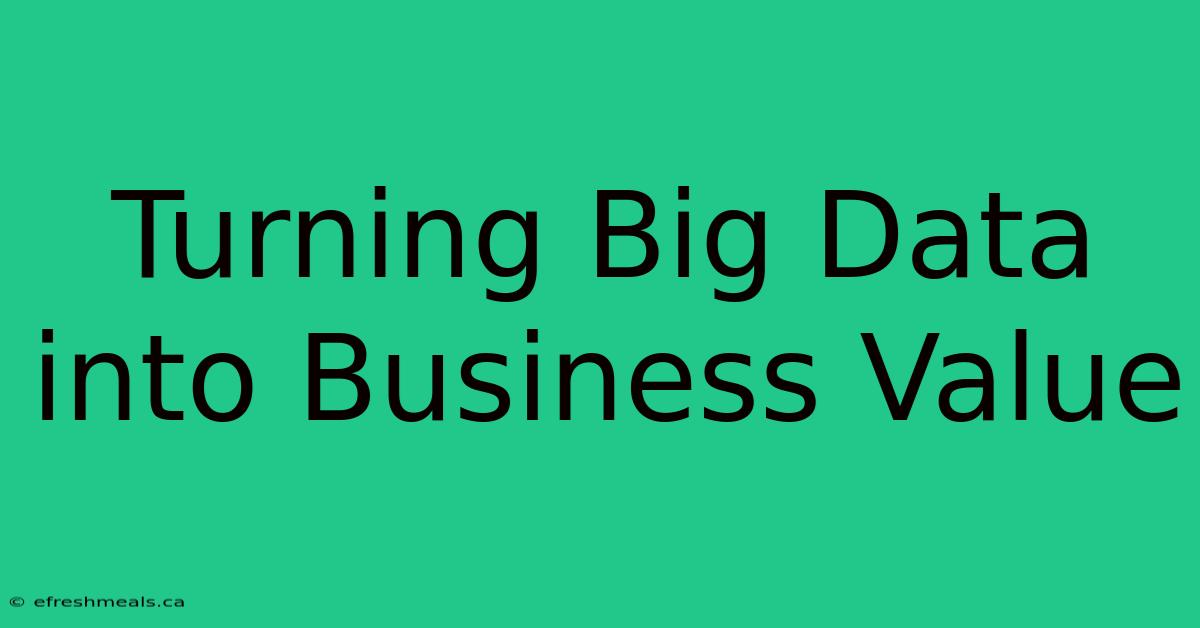
Thank you for visiting our website wich cover about Turning Big Data Into Business Value. We hope the information provided has been useful to you. Feel free to contact us if you have any questions or need further assistance. See you next time and dont miss to bookmark.
Featured Posts
-
Remembering Silvia Pinal A Legacy Remembered
Nov 30, 2024
-
17 000 Spectators At Wwe Survivor Series 2024
Nov 30, 2024
-
Taylor Swift Joins Kelce At Nfl Game
Nov 30, 2024
-
Notre Dame Macron On Cathedrals Reopening
Nov 30, 2024
-
Canadas Economic Slowing The Daily Chase
Nov 30, 2024